Klawonn, F and Höppner, F and Jayaram, Balasubramaniam
(2015)
What are Clusters in High Dimensions and are they Difficult to Find?
In:
Clustering High--Dimensional Data: First International Workshop, CHDD 2012, Naples, Italy, May 15, 2012, Revised Selected Papers.
Lecture Notes in Computer Science, 7627
.
Springer Berlin Heidelberg, pp. 14-33.
ISBN 978-3-662-48576-7
![[img]](http://raiithold.iith.ac.in/2127/1.hassmallThumbnailVersion/2127_clusters_in_high_dimensions.pdf) 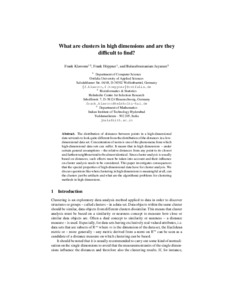 Preview |
|
Text (Author version pre-print)
2127_clusters_in_high_dimensions.pdf
- Accepted Version
Download (629kB)
| Preview
|
Abstract
The distribution of distances between points in a high-dimensional data set tends to look quite different from the distribution of the distances in a low-dimensional data set. Concentration of norm is one of the phenomena from which high-dimensional data sets can suffer. It means that in high dimensions – under certain general assumptions – the relative distances from any point to its closest and farthest neighbour tend to be almost identical. Since cluster analysis is usually based on distances, such effects must be taken into account and their influence on cluster analysis needs to be considered. This paper investigates consequences that the special properties of high-dimensional data have for cluster analysis. We discuss questions like when clustering in high dimensions is meaningful at all, can the clusters just be artifacts and what are the algorithmic problems for clustering methods in high dimensions.
Actions (login required)
 |
View Item |